CDIO 2017 #Assignment 2 Technological
# Different types of technology in autonomous vehicle
Autonomous vehicles are packed with technology. There is a wide variety of sensing and control technology used, including cameras, lidar, GPS, infrared, radar, sensor fusion. The figure below gives a general idea of main autonomous driving technology in the perspective of software and system. There are eight main aspects: Location Technology, Self-Driving Car Maps, Wireless Updates, Cybersecurity, Robotics, Drones, Machine Vision and AI and Neural Networks. Other relevent technology also includes sensor fusion, control strategy, human factor modeling, cloud computation, Human Machine Interaction and so on.
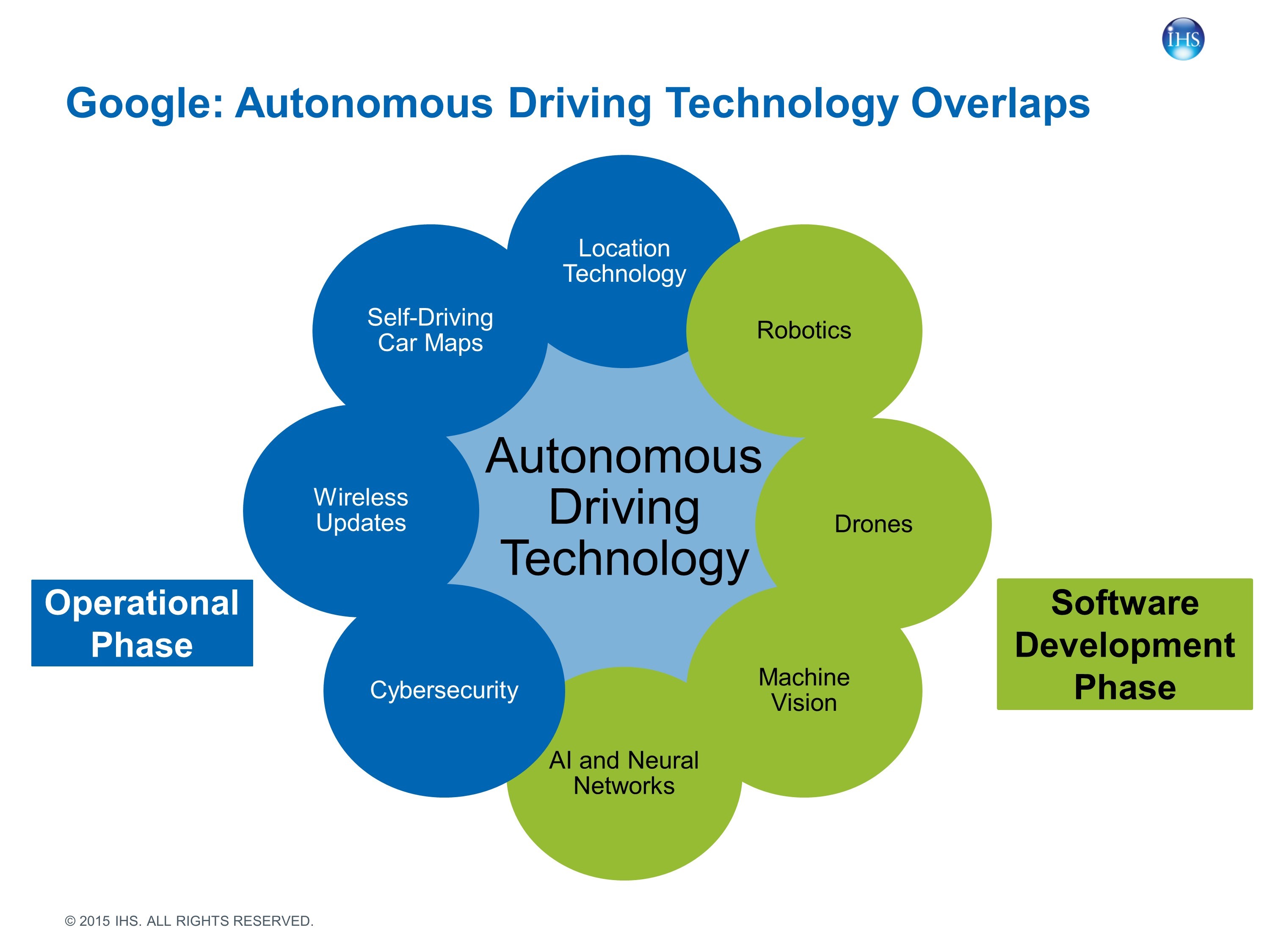
# Sensing Technology
To accomplish environment monitoring, there are various sensor options including LIDAR, camera, Radar. The figure below shows various sensors amounted on vehicle for different purposes. In order to correlate sensors information to work more efficiently and decrease cost, sensor fusion is significant in autonomous driving system. By fusing data from various sources, the estimation of vehicle state and environment monitoring could be more accurate for algorithm to make more correct decisions. For instance, camera is good at recognizing object but less accurate in range sensing, while radar is good at range sensing but unable to recognize objects. Combining these two sensors, vehicle could easily know the exact object is and with accurate posion Meanwhile, redundant sensors are required to guarantee the security of system operation when a few sensors do not work appropriately. Currently, in addition to study sensors to make them more accurate and have higher performance, a lot of OEM and suppliers is working on fusing various sensors.

# Location and Mapping Technology
Modern self-driving cars generally use Bayesian Simultaneous localization and mapping (SLAM) algorithms, which fuse data from multiple sensors and an off-line map into current location estimates and map updates. SLAM with detection and tracking of other moving objects (DATMO), which also handles things such as cars and pedestrians, is a variant being developed by research at Google . Simpler systems may use roadside real-time locating system (RTLS) beacon systems to aid localisation. Typical sensors include LIDAR and stereo vision, GPS and IMU. Visual object recognition uses machine vision including neural networks[1]. Figure below shows SLAM map based on LIDAR, stereo camera and GPS from google autonomous driving project.
# V2X Communication Technology

# Cybersecurity Technology
As we discussed in previous session, cybersecurity is largest threat facing by connected autonomous vehicle. One possible solution to enable vehicle to have several layers of defense. Starting at the foundation, defensive software solutions can be housed locally on individual ECUs. Moving up a level, software can protect the vehicle’s internal network as a whole by examining all network communications, flagging any changes in standard in-vehicle network behavior and stopping attacks from advancing in the network.Next, solutions exist to defend the particular electronic units in a vehicle that are connected to the outside world — for instance, infotainment units. This is a critical layer in the overall cybersecurity defense system, because it represents the border between the vehicle’s internal network and the external world.Finally, cloud security services can detect and correct threats before they reach the vehicle. They also can send the vehicle over-the-air updates and intelligence in real time. In addition to these layers of protection directly relating to a vehicle’s connectivity, supply chain risk management is a critical element of the overall cybersecurity effort. Compromised physical components can jeopardize the integrity of a car’s security architecture, making it imperative that OEMs only source parts from trusted suppliers[3].
# Machine Vision Technology
Machine Vision Technology in context of autonomous driving mainly deal with the object identification and environment detection by SLAM we discussed previously. Relatively mature technology of roadline following mostly relies on machine vision. Or pedestrian detection system uses radar to measure distance forward and camera to capture image to identify pedestrian. With machine learning and optimization algorithm, the identification accuracy could be guaranteed even in noisy environment. Except from vision-based environment detection, machine vision technology largely correlates to environment model reconstruction and artificial intelligence.
# Artificial Intelligence Technology
Intelligence is the brain of vehicle to determine which operation the vehicle execute next. A number of researches nowadays focuses on mimic of human brain to machine therefore vehicle has capability to make more intelligent decision based on environment like experienced driver. Like human brain, vehicle "brain" could learn from previous driving experience or others experience to tackle with critical situation even better than human. Certainly, complete mimic of driving capacity on machine still has long way to go but it is rapidly developing and evoluting.
[1] https://en.wikipedia.org/wiki/Autonomous_car
[1] https://en.wikipedia.org/wiki/Autonomous_car
[2] Vehicle-to-vehicle (V2V) and vehicle-to-infrastructure (V2I) communication in a heterogeneous wireless network – Performance evaluation, K. Chandra, A. Rayamajhi, Transportation Research Part C: Emerging Technologies, Volume 68, P168-184.
[3] https://techcrunch.com/2016/08/25/the-biggest-threat-facing-connected-autonomous-vehicles-is-cybersecurity/
[3] https://techcrunch.com/2016/08/25/the-biggest-threat-facing-connected-autonomous-vehicles-is-cybersecurity/
Please include sources for your images (as they are great and other students may be interested in looking at them!). Cybersecurity is a very interesting topic surrounding autonomous vehicles. - The CDIO Academy Team
ReplyDelete